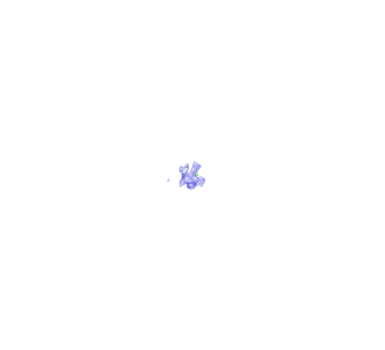
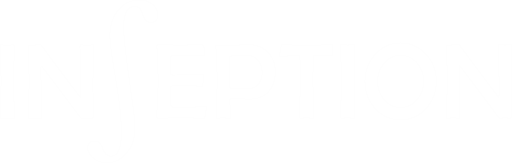
IN Silico research for Estimating Patient’s Tumor evoluTION
Our Vision
A large amount of research work together with recent advances in technology including novel imaging techniques and high resolution genomic studies advocate that tumors are incredibly complex, highly heterogeneous, self-organized biological systems spanning multiple temporal and spatial scales. More specifically, tumors involve processes at i) microscopic level where molecular interactions like signaling and metabolic reactions occur, ii) at cellular and mesoscopic level where cells interact with each other and their local microenvironment, and iii) extend further to tissue-level dynamics. The involved biological processes are strongly coupled and the challenge is to appropriately incorporate them into a complete description, utilizing the available knowledge and data to better understand and predict how tumors evolve.
Because of the complexity and diversity of the mechanisms involved in tumor evolution, mathematical and computational approaches (a.k.a. in silico) aiding in our understanding, become inevitable. We postulate that the construction of a faithful, patient-specific mathematical model that incorporates the underlying mechanisms and the involved interactions and heterogeneity could explain the potential outcomes and provide guidelines for optimal therapy planning, better understanding of tumor pathophysiology and dictate future experiments.
In this direction, the INSEPTION group adopts the Systems Biology strategy to improve understanding of cancer complexity and increase predictive power with personalized clinical relevance, combining novel biological experiments, imaging techniques and advanced in silico methods. The INSEPTION group argues that cancer can only be deeply understood and cured through the collaboration among scientists from different disciplines that could potentially bridge the gap between basic science and the clinic.
“What I cannot (re)create,
I do not understand.”
Our news
Brain Oncology is a complex scientific field that necessitates the collaboration among scientists from different disciplines. We focus on Glioblastoma (GB), a maligant brain tumor with extremely poor prognosis for the patient.
Despite of the rapid advances in technology and novel therapeutics, therapy treatment in GB patient fails mainly due to its extensive inter- and intra-tumoral heterogeneity and its high recurrence potential.
The integration of mathematical and computational approaches with experimental data and advanced imaging techniques is more than important in understanding brain pathophysiology and tumor progression in a patient-specific way.